Speaker Diarization in Transcription: A Powerful Tool for Clarity and Precision
Bei TranscribetoText.ai, we’re committed to delivering the best transcription experience possible, and that includes integrating speaker diarization to make transcripts more accurate and usable. Speaker diarization—sometimes called “speaker recognition” or “speaker attribution”—is the process of identifying and separating speakers within an audio file. This feature is critical for creating transcripts that not only capture what’s being said but also clarify who’s saying it.
Why Speaker Diarization Matters in Transcription
In any scenario involving multiple speakers, it can quickly become challenging to follow the flow of conversation in a text document. Speaker diarization helps by marking each speaker’s turn in the conversation, which is especially useful for meetings, interviews, panel discussions, and customer service recordings. Without this, a transcription might look like a single monologue, making it hard to distinguish who’s talking and leading to confusion, particularly in critical discussions with differing viewpoints.
Speaker attribution enables readers to follow a transcript as they would a live conversation, identifying contributions and intentions more easily. This functionality becomes essential in scenarios where distinguishing between speakers is critical, such as legal transcriptions, academic research, media interviews, and customer feedback analysis. By recognizing distinct voices and linking spoken content to individual speakers, we create transcriptions that can be directly referenced, quoted, or analyzed with confidence.
How Speaker Diarization Works on TranscribetoText.ai
With our tool, you can choose to recognize between two and eight speakers, based on the audio’s complexity. Unlike other transcription tools that limit or automate speaker identification, TranscribetoText.ai gives you control. Setting the number of speakers improves accuracy, especially when speakers have similar tones.
Our service uses OpenAI’s Whisper model, one of the most advanced transcription and recognition models on the market today. Whisper is built for high accuracy and is particularly adept at managing various dialects, accents, and languages. Whisper’s performance in speaker diarization surpasses many traditional models, thanks to its advanced machine learning techniques that understand context and differentiate speakers even in challenging audio conditions, such as overlapping speech or background noise.
Benefits of Using Whisper for Diarization
The Whisper model shines when handling complex audio environments, where traditional models often fall short. Unlike simpler models, Whisper can recognize subtle tonal differences and detect when a speaker changes, providing higher accuracy in differentiating voices. This is particularly beneficial in business meetings, where clarity is crucial for decision-making, or in legal proceedings, where accurate speaker attribution is necessary for evidence.
The strength of Whisper in managing noise, varied speech patterns, and accent diversity is unmatched. For TranscribetoText.ai users, this means that transcriptions aren’t just accurate—they’re exceptionally reliable, even in complex scenarios. Whisper’s ability to handle multiple languages also means that our service can cater to multilingual recordings, an important feature for global organizations or teams working in diverse linguistic contexts.
Real-World Applications of Speaker Diarization
- Corporate Meetings
In large corporate settings, meeting transcripts are invaluable for tracking decisions, recording action points, and maintaining transparency. Diarization ensures that individual contributions are clearly identified, which can be helpful for follow-ups and accountability. Being able to refer to a transcript that accurately tags each participant’s statements saves time and minimizes misunderstandings, particularly in team settings where various departments or roles are involved. - Media Interviews
Journalists and researchers frequently conduct interviews where identifying each speaker’s input is essential. In interviews, the ability to attribute each statement to the correct speaker provides a factual, unaltered record of events. For example, in a press interview with multiple spokespersons, speaker diarization ensures that the transcript accurately reflects who said what, maintaining the integrity of each individual’s comments. - Customer Service Recordings
Customer interactions can often involve complex dialogues between support staff and customers. Diarization allows organizations to analyze interactions for quality control, training, and customer satisfaction purposes. By identifying each party in the conversation, customer service teams can gain clearer insights into pain points, agent performance, and the nuances of customer complaints. - Focus Groups and Research Discussions
For market researchers, accurately capturing the voice of each participant in a focus group is invaluable. Diarization allows researchers to analyze responses individually, ensuring that insights are attributed to the correct participants. This accuracy helps researchers identify trends, unique perspectives, and demographic-specific feedback, enhancing the quality of their analyses and reports.
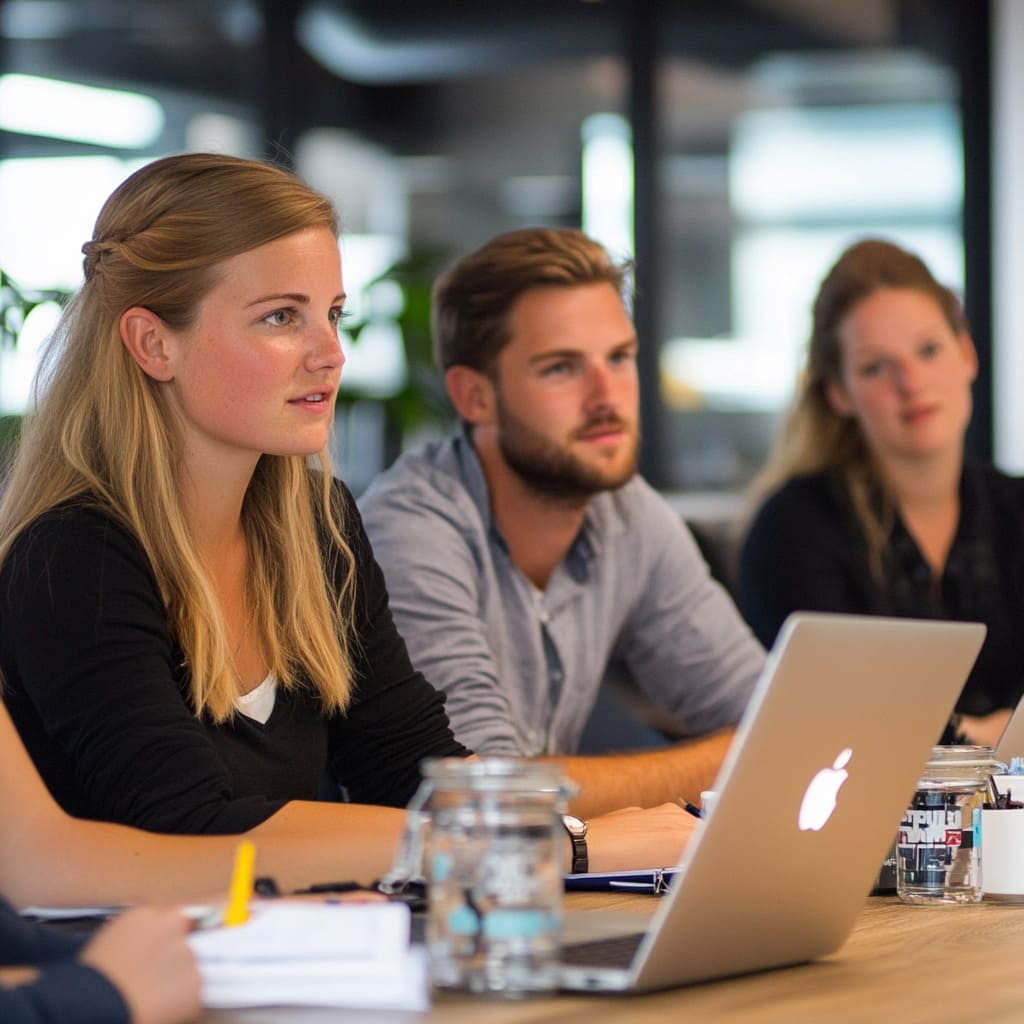
Tips for Using Speaker Diarization Effectively
- Set Clear Speaker Numbers
One of the strengths of TranscribetoText.ai’s diarization feature is the option to choose the number of speakers, from two to eight. Setting an accurate speaker count improves recognition accuracy. For example, in a panel discussion with four known participants, setting the speaker count to four helps the model tailor its processing to the right number of voices, reducing the likelihood of misattribution. - Use High-Quality Audio
Diarization, like all transcription, benefits greatly from good audio quality. Background noise, overlapping speech, and unclear audio can affect the diarization process. When possible, ensure that audio is recorded in a quiet environment and use quality recording equipment. This helps the Whisper model perform at its best, delivering clearer speaker segmentation and reducing potential confusion. - Select TranscribetoText.ai’s Speaker Labeling Options
In our platform, users can label speakers as “Speaker 1,” “Speaker 2,” etc., or customize labels post-transcription to reflect real names or titles. This feature is helpful for organizational clarity, especially if the transcript will be used by a wider team or in formal documentation. Renaming speakers with actual names or roles after transcription can help in tracking discussions more intuitively. - Review and Edit
While Whisper provides high accuracy, some scenarios may require minor adjustments to ensure the transcript’s accuracy. A quick review can confirm speaker attributions, allowing you to catch and correct any misattributions swiftly. The user-friendly editing tools on TranscribetoText.ai make it easy to make these final touches before exporting or sharing the transcript.
Enhancing Diarization with AI Advancements
At TranscribetoText.ai, we’re constantly evolving our platform to include cutting-edge AI improvements, keeping us at the forefront of transcription technology. Our partnership with OpenAI’s Whisper model provides our users access to state-of-the-art speaker recognition capabilities, setting a new standard for transcription accuracy and usability. As the industry advances, so will our tools, ensuring that our users always have access to the best available technology for speaker diarization.